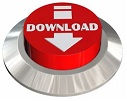
Then we could proceed to generate the corresponding power curves and make valid comparisons. This would be done, in our Monte Carlo experiment, by “jiggling” the critical values used for the t-test to ones that ensure that the test has a 5% rejection rate when the null is true. One way to deal with this is to “size-adjust” any test that exhibits size distortion. In all other cases where there is size distortion, the “true” power will not be clear. Then, Test A is more powerful than Test B.
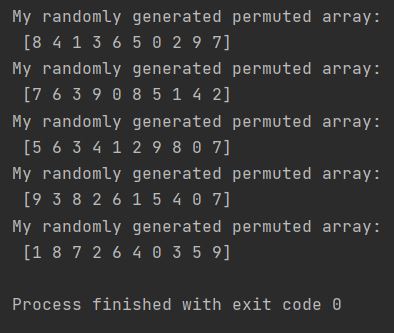
PERMUTE RANDOM ROPE DEFINE FULL
The only exception is when Test A has lower actual significance level than Test B, but Test A has a higher rejection rate than Test B when the null is false ( i.e., higher “raw”, or apparent, power”) over the full parameter space. Strictly, power comparisons are valid when the tests have the same (actual, empirical) significance level.
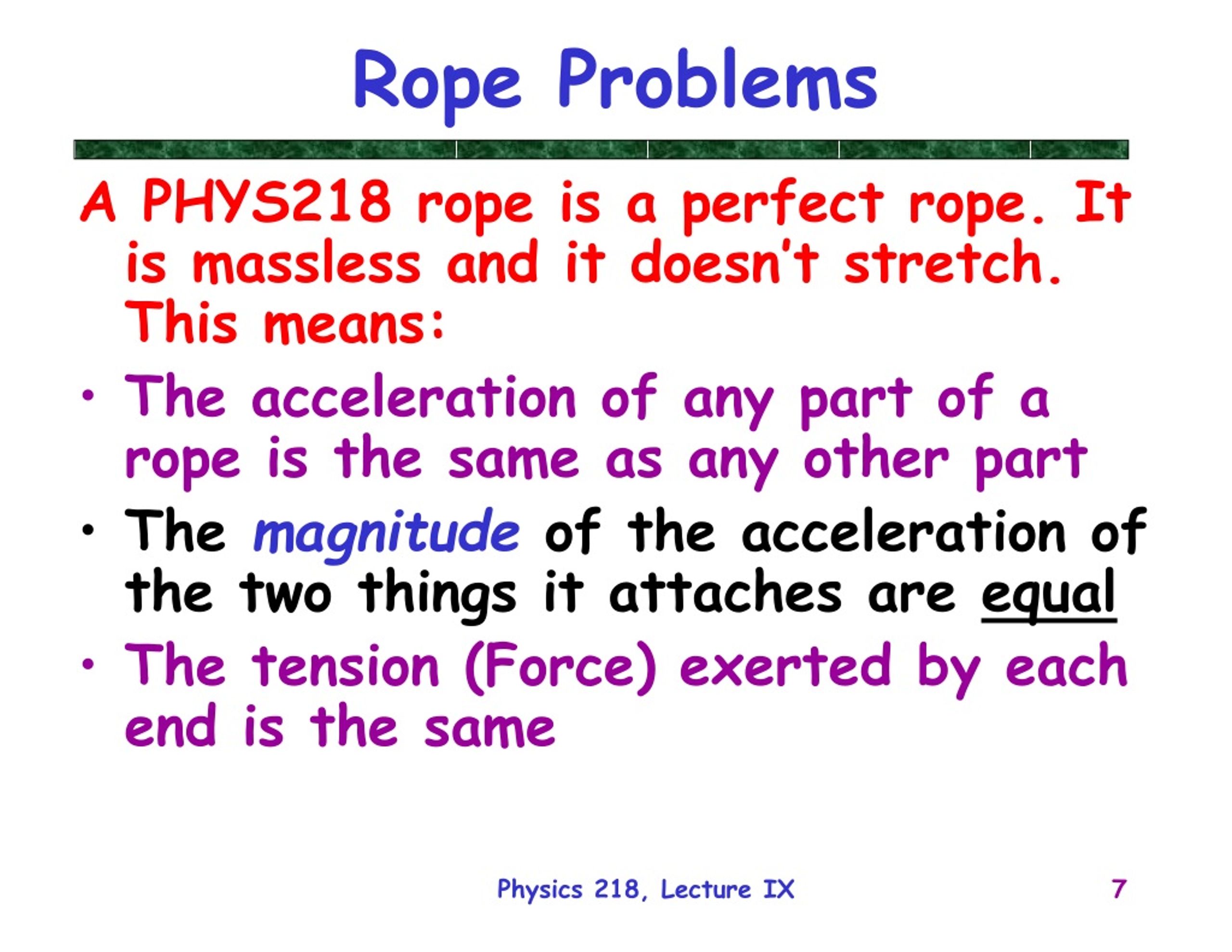
We need to be careful, here, when we talk about comparisons between the powers of the two tests (and this comment is universally applicable). The magnitudes of the size distortions and the powers are specific to this choice.Ģ. This chosen value for β 1 affects the (numerical) results. We set β 1 = 1 in the DGP and this resulted in a mis-specified “fitted” regression model. The second ( really neat) thing that we see in the second line of that table is that the permutation test still has a significance level of 5%! Even though the model is mis-specified, this doesn’t affect the test – at least in terms of it still being “exact” with respect to the significance level that we wanted to achieve.ġ. We wanted to apply the test at the 5% significance level, but in fact it only rejected the null, when it was true, 1% of the time! Of course, if we just mis-applied this test once, in an application, we would have no idea if there was any substantial size-distortion or not. First, the t-test has a downwards size-distortion. In the second line of Table 1 we see two important things. The extent of this difference is the “size-distortion” associated with the test when we mis-apply it in this way. Depending on the situation, it may be less than 5%, or greater than 5%. Obviously, the usual (central) Student-t critical values are no longer correct, and the observed significance level (the rejection rate of the null in the experiment when the null is true) will differ from 5%. It’s value is unobservable! And w e can’t use the critical value(s) from the non-central t distribution if we don’t know the value of the non-centrality parameter. In this case the usual t-statistic follows a non-central Student-t distribution, with a non-centrality parameter that increases monotonically with β 1 2, and which depends on the x data and the variance of the error term. We’ve omitted a (constant) regressor from the estimated model. However, now, in Table 1, β 1 = 1, so the DGP includes an intercept and the fitted model is under-specified. Recall that the estimated model omits the intercept – the model is fitted through the origin. Let’s take a look back at Table 1, and now focus on the second line of results (highlighted in orange). So far, all that we seem to have shown is that the permutation test and the t-test exhibit no “size-distortion” when the model is correctly specified, and the errors satisfy the assumptions needed for the t-test to be valid. More formally, using the uniftest package in R we find that the Kolmogorov-Smirnov test statistic for uniformity is D = 0.998 (p = 0.24) and the Kuiper test statistic is V = 1.961 (p = 0.23).
PERMUTE RANDOM ROPE DEFINE CODE
One point that I covered was that if the null hypothesis is true, then this sampling distribution has to be Uniform on, regardless of the testing problem! This result gives another way of checking if the code for our simulation experiment is performing accurately, and that we’ve used enough replications and random selections of the permutations.Īs we can see, this distribution is “reasonably uniform”, as required. In an old post on this blog I discussed the sampling distribution of a p-value. we could obtain the 5% rejection rate correctly, even if the distribution of the p-values from which this rate was calculated is “weird”. However, the result for the randomization test could be misleading. Given the particular errors that were used in the DGP in the simulations, this had to happen for the t-test. Notice that the reported empirical significance levels match the anticipated 5%! That is, the DGP in (1) has no intercept, so the model is correctly specified, and the null hypothesis of a zero slope is true.īecause the null hypothesis is true, the power of the test is just its significance level (5%).
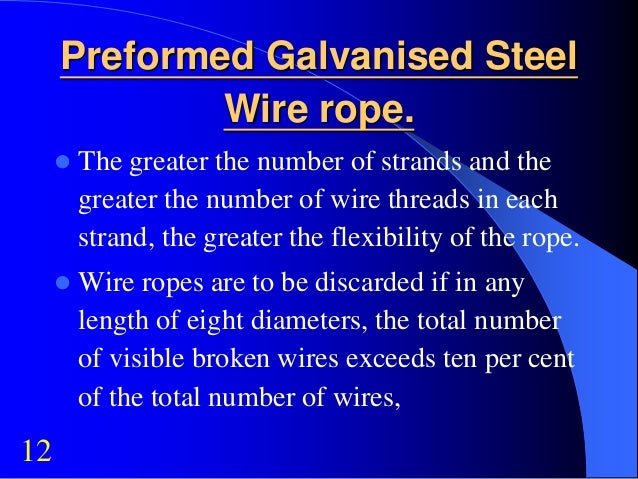
This corresponds to the case where both β 1 and β 2 are zero. We’ll come back to this table shortly, but for now just focus on the row that is highlighted in light green.
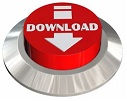